In today’s digital landscape, where cyberattacks are becoming increasingly sophisticated, securing our data and systems has never been more critical. Traditional security measures are struggling to keep pace with the ever-evolving threat landscape. This is where machine learning steps in, offering a powerful and adaptive solution to bolster cybersecurity defenses.
By leveraging the power of artificial intelligence, machine learning algorithms can analyze vast amounts of data, identify patterns, and detect anomalies that might otherwise go unnoticed. This allows for proactive threat identification, prevention, and response, giving organizations a significant edge in the fight against cybercriminals. Join us as we explore the transformative impact of machine learning in cybersecurity and discover how this dynamic alliance is revolutionizing the way we protect our digital assets.
Understanding the Cybersecurity Landscape
The cybersecurity landscape is constantly evolving, becoming increasingly complex and challenging. With the rapid growth of digital technologies, cybercriminals are constantly finding new ways to exploit vulnerabilities and breach networks. This has led to a surge in sophisticated cyberattacks, ranging from data breaches and ransomware to phishing scams and denial-of-service attacks.
Traditional security measures, such as firewalls and intrusion detection systems, are often struggling to keep pace with the evolving threat landscape. Cybercriminals are leveraging advanced techniques like artificial intelligence (AI) and machine learning (ML) to automate their attacks and evade detection.
To effectively combat these threats, organizations need to adopt a more proactive and intelligent approach to cybersecurity. This is where machine learning comes in.
The Role of Machine Learning in Cybersecurity
In today’s digital landscape, cybersecurity is paramount. As cyber threats evolve and become increasingly sophisticated, traditional security measures are often insufficient. This is where machine learning (ML) emerges as a powerful ally, revolutionizing cybersecurity by providing automated and intelligent solutions.
ML algorithms can analyze vast amounts of data to identify patterns and anomalies that might indicate malicious activity. This ability to detect subtle deviations from normal behavior is crucial for early threat detection. By learning from historical data, ML models can predict future attacks and proactively implement protective measures.
One of the key roles of ML in cybersecurity is intrusion detection and prevention. ML-powered systems can continuously monitor network traffic and identify suspicious activities that might go unnoticed by human analysts. They can also differentiate between legitimate and malicious traffic, preventing attacks before they cause damage.
Another significant application of ML is in phishing detection. By analyzing the content, structure, and sender information of emails, ML algorithms can identify phishing attempts with high accuracy. This helps prevent users from falling victim to social engineering attacks and protects sensitive data.
Moreover, ML plays a vital role in endpoint security. By analyzing data from endpoints like laptops, smartphones, and tablets, ML models can detect and block malware, ransomware, and other threats in real time. This helps to safeguard individual devices and prevent the spread of infections across an organization’s network.
In addition to these specific applications, ML also contributes to overall cybersecurity by automating tasks, improving threat intelligence, and enhancing incident response capabilities. It allows security professionals to focus on strategic initiatives while ML handles the tedious and repetitive tasks, freeing up valuable time and resources.
However, it’s essential to acknowledge that ML is not a silver bullet. Data quality is crucial for effective ML-based security solutions. Biased or incomplete data can lead to inaccurate predictions and false alarms. It’s also crucial to ensure that ML models are regularly updated to adapt to the ever-evolving threat landscape.
In conclusion, machine learning is an indispensable tool in the fight against cybercrime. Its ability to analyze massive datasets, identify anomalies, and predict threats enables organizations to stay ahead of the curve and secure their digital assets. As ML technology continues to advance, its role in cybersecurity will only grow stronger, shaping a future where digital protection is more intelligent and proactive than ever before.
Benefits of Using Machine Learning for Cybersecurity
Machine learning (ML) is rapidly transforming the cybersecurity landscape, offering a powerful new weapon in the fight against cyber threats. By leveraging the power of algorithms to analyze vast amounts of data and identify patterns, ML can detect and respond to threats in real-time, providing significant benefits for organizations.
Enhanced Threat Detection
Traditional security solutions often struggle to keep up with the ever-evolving nature of cyberattacks. ML algorithms can analyze massive datasets of network traffic, user behavior, and system logs to identify anomalies and potential threats that may be missed by human analysts. This allows for early detection of threats, giving organizations a crucial head start in mitigating potential damage.
Improved Security Posture
ML can be used to proactively identify security vulnerabilities and weaknesses in systems and networks. By analyzing data from various sources, ML algorithms can pinpoint areas where security measures are lacking, allowing organizations to strengthen their defenses and prevent attacks from exploiting weaknesses. This can include identifying outdated software, misconfigured systems, and other security vulnerabilities.
Automated Response and Mitigation
ML algorithms can be trained to automatically respond to threats in real-time, without human intervention. This can include blocking malicious traffic, isolating infected systems, and triggering incident response protocols. Automated response capabilities not only speed up the mitigation process but also reduce the risk of human error, ensuring faster and more effective protection.
Reduced False Positives
Traditional security solutions often generate a high number of false positives, creating unnecessary alerts and diverting security teams’ attention. ML algorithms can be trained to identify patterns associated with genuine threats, reducing the number of false positives and improving the accuracy of security alerts.
Scalability and Efficiency
As cyber threats grow increasingly complex and sophisticated, security teams struggle to keep up with the workload. ML-powered solutions can automate many of the tasks traditionally handled by human analysts, allowing security teams to focus on more strategic activities and improve overall efficiency.
Overall, machine learning offers numerous benefits for cybersecurity. By leveraging the power of algorithms to analyze data, detect threats, and automate responses, organizations can enhance their security posture, reduce the risk of attacks, and protect their valuable assets.
Common Use Cases of Machine Learning in Cybersecurity: Threat Detection
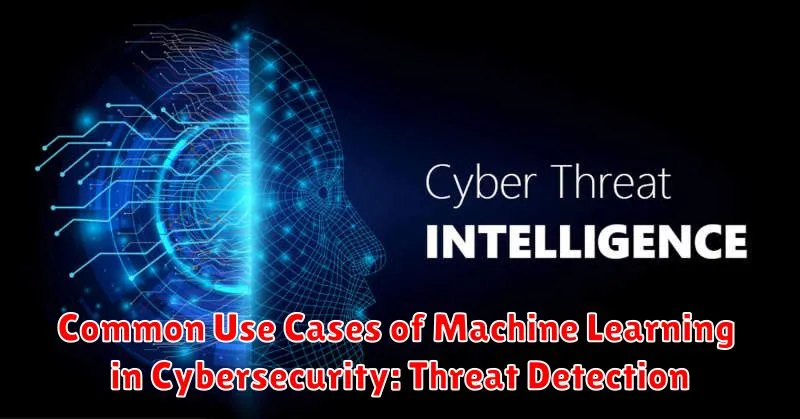
Machine learning (ML) is rapidly changing the cybersecurity landscape, offering powerful tools for enhancing threat detection and response. By analyzing vast amounts of data and identifying patterns, ML algorithms can detect anomalies and potential threats that may go unnoticed by traditional security measures. Here are some common use cases of ML in cybersecurity for threat detection:
1. Intrusion Detection Systems (IDS): ML-powered IDSs can analyze network traffic in real-time, identifying suspicious patterns and behaviors that may indicate an intrusion attempt. These systems can learn from past attacks and adapt to evolving threats, making them more effective at detecting zero-day attacks.
2. Malware Detection: ML algorithms can analyze file characteristics, behavior patterns, and code structures to identify malicious software. By learning from known malware samples, ML models can detect new and unknown threats with high accuracy.
3. Phishing Detection: ML can analyze email content, sender details, and other factors to identify phishing attempts. By recognizing patterns in phishing emails, ML models can flag suspicious messages and protect users from falling victim to social engineering attacks.
4. Anomaly Detection: ML algorithms can detect unusual activities within networks and systems, identifying potential threats that may not be easily recognized by traditional security tools. This approach can be particularly effective in detecting insider threats and other forms of advanced attacks.
5. Security Information and Event Management (SIEM): ML can enhance SIEM systems by automatically correlating events, identifying security incidents, and prioritizing alerts. This allows security teams to focus on the most critical threats and respond more efficiently.
By leveraging these use cases, organizations can significantly strengthen their security posture and enhance their ability to detect and respond to threats in a timely and efficient manner.
Common Use Cases of Machine Learning in Cybersecurity: Intrusion Prevention
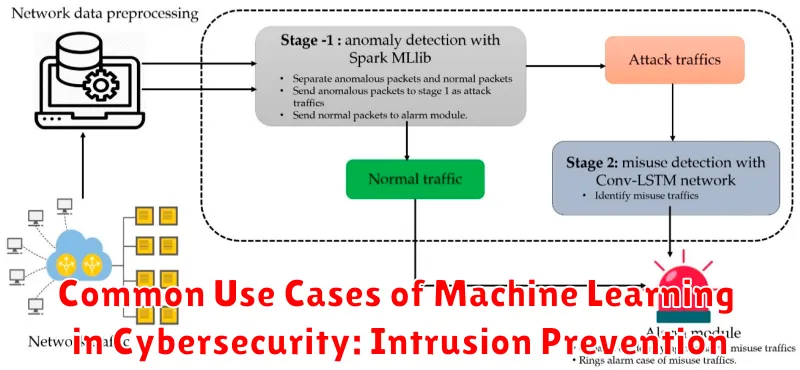
Machine learning (ML) is revolutionizing the cybersecurity landscape by empowering organizations to proactively identify and mitigate threats. One of the most impactful applications of ML in cybersecurity is intrusion prevention. By analyzing vast amounts of data, ML algorithms can learn patterns and anomalies associated with malicious activity, enabling the detection and blocking of intrusions before they cause damage.
Here are some common use cases of ML for intrusion prevention:
1. Network Intrusion Detection and Prevention
ML algorithms can analyze network traffic to detect deviations from normal patterns, such as unusual communication protocols, suspicious data packets, and abnormal traffic volumes. This enables the identification of potential attacks and the blocking of malicious traffic in real-time.
2. Endpoint Security
ML is used to detect malicious software, malware, and suspicious behavior on endpoints, such as computers and mobile devices. By analyzing system calls, file activity, and network connections, ML algorithms can identify threats that traditional antivirus software might miss.
3. Anomaly Detection
ML algorithms can be trained to identify anomalies in system logs, user behavior, and other data sources. By recognizing deviations from expected patterns, security teams can detect unusual activities that may indicate a compromise.
4. Behavioral Analytics
ML can analyze user behavior patterns to identify potential threats. By tracking user activity, such as login attempts, file access, and network connections, ML algorithms can detect anomalies and flag suspicious actions. This helps prevent unauthorized access and data breaches.
In conclusion, ML is a powerful tool for intrusion prevention, providing organizations with enhanced visibility and proactive defense capabilities. By leveraging the power of ML, security teams can effectively detect and block threats, minimizing the risk of cyberattacks.
Common Use Cases of Machine Learning in Cybersecurity: Malware Analysis
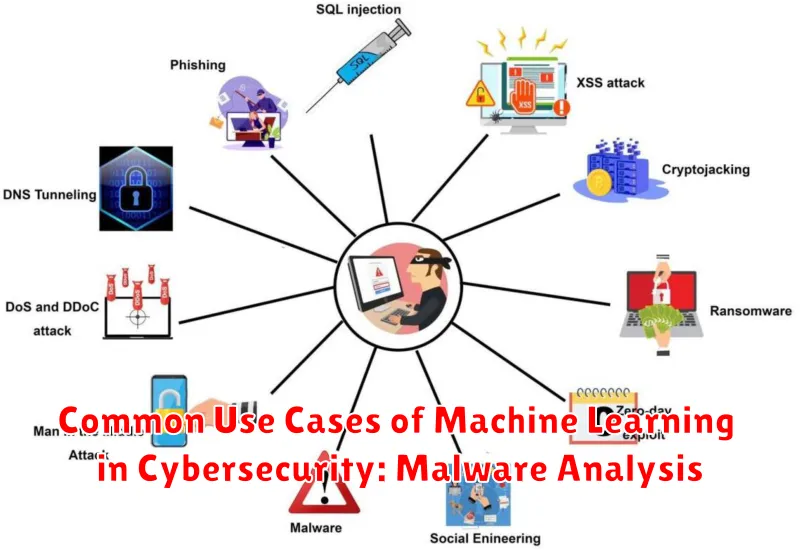
Malware analysis is a crucial aspect of cybersecurity, as it helps identify, understand, and mitigate the threats posed by malicious software. Machine learning (ML) has emerged as a powerful tool in this domain, offering several advantages over traditional methods. Here are some common use cases of ML in malware analysis:
1. Malware Detection: ML algorithms can be trained on massive datasets of known malware samples to learn patterns and characteristics associated with malicious code. This allows for the identification of new and unknown malware variants, even those that have never been seen before. By analyzing features like file structure, API calls, and code behavior, ML models can detect suspicious activity and flag potential threats.
2. Malware Classification: ML models can classify malware into different categories based on their functionality, such as ransomware, trojans, or worms. This classification can help security teams prioritize responses and implement appropriate mitigation strategies. For instance, knowing the specific type of malware can guide the selection of appropriate anti-malware software or the development of targeted threat intelligence.
3. Sandboxing and Behavioral Analysis: ML can enhance sandboxing techniques by analyzing the behavior of suspicious files in a controlled environment. ML models can learn from past malware behavior to identify unusual or suspicious actions, allowing for faster and more accurate detection of malicious intent. This can help distinguish benign software from malicious code that might mimic legitimate behavior.
4. Malware Attribution: ML can aid in attributing malware attacks to specific groups or individuals. By analyzing the code, communication patterns, and other technical characteristics of malware, ML models can identify patterns that link different attacks to the same source. This information is crucial for understanding the threat landscape and taking proactive measures against targeted attacks.
5. Dynamic Analysis: ML can be applied to dynamic analysis, which involves monitoring the behavior of software while it’s executing. By analyzing the interactions of malware with the system and other applications, ML models can identify malicious activities, even if they are hidden from static analysis. This dynamic approach provides a more comprehensive understanding of malware behavior.
In conclusion, ML offers significant benefits for malware analysis, enabling faster detection, accurate classification, and deeper understanding of malicious threats. As the threat landscape continues to evolve, the integration of ML into cybersecurity solutions will become increasingly critical for defending against emerging malware threats.
Common Use Cases of Machine Learning in Cybersecurity: Phishing Detection
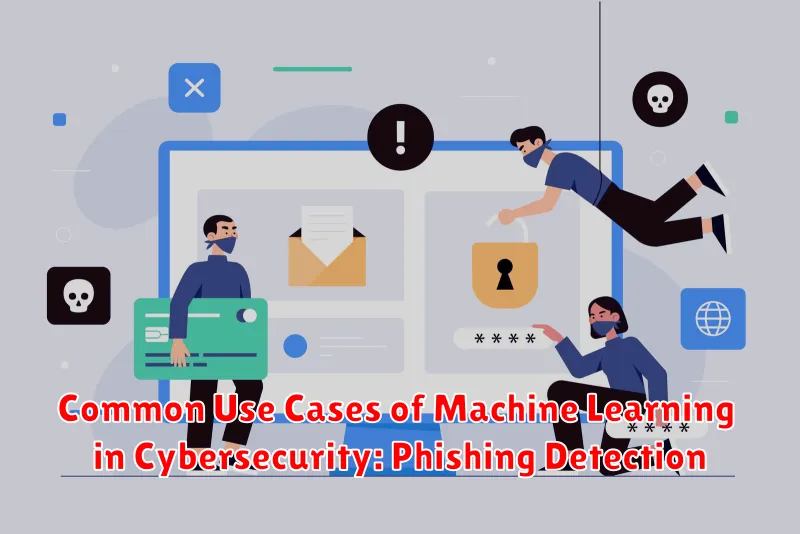
Phishing attacks are a major threat to individuals and organizations alike. These attacks often involve malicious emails or websites designed to trick users into revealing sensitive information, such as login credentials or credit card details. Machine learning is proving to be a valuable tool in the fight against phishing.
One of the most common use cases of machine learning in cybersecurity is phishing detection. By analyzing large datasets of emails and websites, machine learning algorithms can identify patterns and anomalies that are indicative of phishing attacks. For instance, algorithms can learn to recognize suspicious email subject lines, unusual links, and grammatical errors that are often present in phishing emails.
Machine learning can also be used to detect phishing attacks in real-time. By monitoring user behavior and network traffic, algorithms can flag suspicious activity and prevent attacks before they can succeed. This real-time detection is crucial for protecting users from the latest phishing threats, which are constantly evolving.
In addition to detecting phishing attacks, machine learning can also be used to improve the accuracy of phishing detection systems. By continuously learning from new phishing attacks, algorithms can become more effective at identifying and blocking future attacks. This continuous learning process helps to ensure that phishing detection systems remain effective against the ever-changing threat landscape.
Overall, machine learning is playing a critical role in enhancing cybersecurity by improving our ability to detect and prevent phishing attacks. As the threat of phishing continues to grow, machine learning will become increasingly important in safeguarding individuals and organizations from these malicious attacks.
Common Use Cases of Machine Learning in Cybersecurity: Security Automation
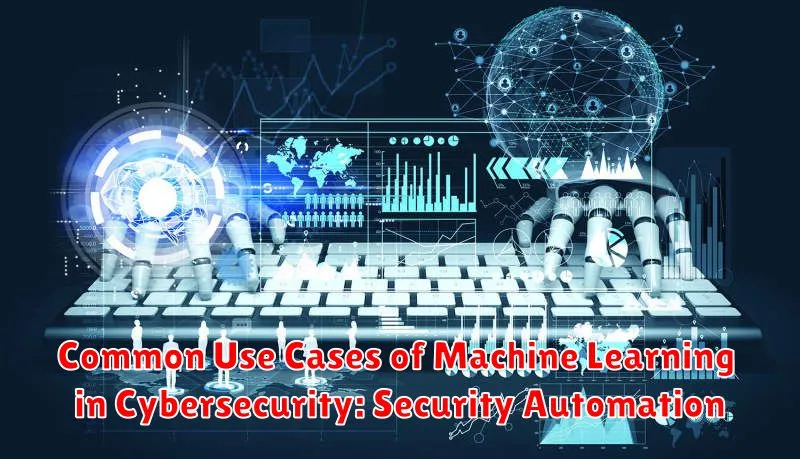
Machine learning (ML) has emerged as a transformative force in cybersecurity, empowering organizations to automate security tasks, enhance detection capabilities, and bolster defenses against evolving threats. ML algorithms can analyze vast amounts of data, identify patterns, and make predictions, enabling automated responses and proactive security measures.
One of the most prominent use cases of ML in cybersecurity is security automation. ML algorithms can automate repetitive and time-consuming security tasks, freeing up security professionals to focus on more strategic initiatives. This includes tasks such as:
- Threat detection and response: ML can analyze network traffic, system logs, and other data sources to identify suspicious activities, anomalies, and potential threats. It can then trigger automated responses, such as blocking malicious IP addresses, isolating infected systems, or sending alerts to security personnel.
- Vulnerability scanning and patching: ML can help identify vulnerabilities in systems and applications, prioritize patching efforts, and automate the deployment of security patches.
- Incident response: ML can analyze incident data to identify patterns, determine the root cause of incidents, and recommend appropriate response actions. This helps expedite incident response and minimize the impact of security breaches.
- User behavior analysis: ML can analyze user activity patterns to detect anomalies that could indicate malicious activity. This can help identify unauthorized access, suspicious login attempts, or data exfiltration attempts.
- Email spam filtering: ML algorithms can learn to identify spam emails based on content, sender, and other characteristics, automatically filtering them out of inboxes.
- Phishing detection: ML can analyze email content and website URLs to identify phishing attempts and prevent users from clicking on malicious links.
By automating these tasks, ML empowers security teams to operate more efficiently, respond to threats more effectively, and strengthen their overall security posture.
Challenges and Limitations of Machine Learning in Cybersecurity
While machine learning offers a powerful arsenal for bolstering cybersecurity, it’s crucial to acknowledge the inherent challenges and limitations that accompany its implementation.
One significant challenge lies in the potential for adversarial attacks. Malicious actors can deliberately manipulate training data to mislead machine learning models, causing them to make incorrect decisions or become vulnerable to exploits. This underscores the importance of robust data validation and defense mechanisms.
Another challenge is the need for large datasets. Training effective machine learning models requires a significant amount of data, which can be challenging to acquire, particularly in the context of emerging threats or niche attack vectors. This can lead to limitations in the model’s ability to generalize to unseen scenarios.
Furthermore, interpretability remains a challenge. While machine learning models can often achieve impressive accuracy, understanding the reasoning behind their predictions can be difficult. This lack of transparency can hinder trust and make it difficult to identify potential biases or weaknesses.
Finally, the ongoing evolution of threats presents a constant challenge for machine learning in cybersecurity. As attackers develop new techniques, models trained on past data may become ineffective. Regular model retraining and adaptation are essential to maintain effectiveness in a dynamic threat landscape.
Despite these challenges, the potential of machine learning in cybersecurity is undeniable. Addressing these limitations through ongoing research, robust security practices, and a collaborative approach is crucial for realizing the full potential of this powerful alliance.
Best Practices for Implementing Machine Learning in Cybersecurity
Machine learning is revolutionizing cybersecurity, offering powerful tools for threat detection, prevention, and response. But its successful implementation requires a careful approach. Here are some best practices for integrating machine learning into your cybersecurity strategy:
1. Define Clear Objectives: Begin by identifying specific cybersecurity challenges you want to address with machine learning. Are you looking to improve threat detection, automate incident response, or enhance vulnerability management? Having clear objectives will guide your model selection, data collection, and evaluation.
2. Gather High-Quality Data: Machine learning models are only as good as the data they are trained on. Ensure you have access to a sufficient volume of relevant and accurate data, including both malicious and benign examples. Data cleansing and preprocessing are essential steps to ensure model reliability.
3. Choose the Right Model: Different machine learning algorithms are suited for different tasks. For example, supervised learning is effective for anomaly detection, while unsupervised learning can be used for clustering and identifying patterns. Consider the nature of your data and the cybersecurity goals you aim to achieve when selecting a model.
4. Implement Robust Evaluation and Testing: Thorough evaluation is crucial to ensure the effectiveness of your machine learning model. Use appropriate metrics and test the model against unseen data to assess its accuracy, precision, and recall. Regular retraining and model updates are essential to maintain performance as threats evolve.
5. Integrate with Existing Systems: Seamlessly integrate your machine learning models with your existing cybersecurity infrastructure. This ensures that insights generated by the models are actionable and can be used to enhance your overall security posture.
6. Ensure Transparency and Explainability: Machine learning models can sometimes produce complex predictions that are difficult to understand. Implement mechanisms for transparency and explainability, allowing security analysts to interpret the model’s output and build trust in its decisions.
7. Address Bias and Ethical Considerations: Machine learning models can inherit biases from the data they are trained on. Be mindful of potential biases and take steps to mitigate them. Consider the ethical implications of your model’s decisions, especially when dealing with sensitive data.
By following these best practices, you can effectively leverage the power of machine learning to enhance your cybersecurity defenses and build a more resilient and proactive security posture.
Future Trends in Machine Learning for Cybersecurity
Machine learning (ML) is rapidly transforming the cybersecurity landscape, offering powerful tools for detecting and preventing threats. As we move forward, several exciting trends are set to shape the future of ML in cybersecurity:
1. Enhanced Threat Detection: ML algorithms will become increasingly sophisticated in identifying complex and evolving threats. Advanced techniques like deep learning and natural language processing will enable systems to analyze vast amounts of data, uncovering hidden patterns and anomalies that traditional methods might miss. This will significantly improve the accuracy and timeliness of threat detection.
2. Automated Security Response: ML-powered systems will automate security response actions, such as blocking malicious traffic or quarantining infected devices. This automation will free up security professionals to focus on more strategic tasks and will allow for faster and more effective threat mitigation.
3. Proactive Security: ML will play a crucial role in proactive security measures. By analyzing historical data, ML models can anticipate potential threats and implement preventive measures before attacks occur. This predictive capability will significantly reduce the risk of breaches and minimize damage.
4. Personalized Security: ML will enable personalized security solutions tailored to the specific needs of individual users and organizations. By analyzing user behavior and network activity, ML systems can identify and address vulnerabilities unique to each entity. This will provide more targeted and effective protection.
5. Explainable AI: The increasing use of complex ML algorithms necessitates the development of explainable AI (XAI) techniques. XAI aims to make ML models more transparent and understandable, allowing security professionals to comprehend the decision-making process and trust the results.
The future of ML in cybersecurity holds immense promise for strengthening defenses against evolving threats. By embracing these trends, organizations can leverage the power of ML to enhance their security posture and protect their valuable assets.